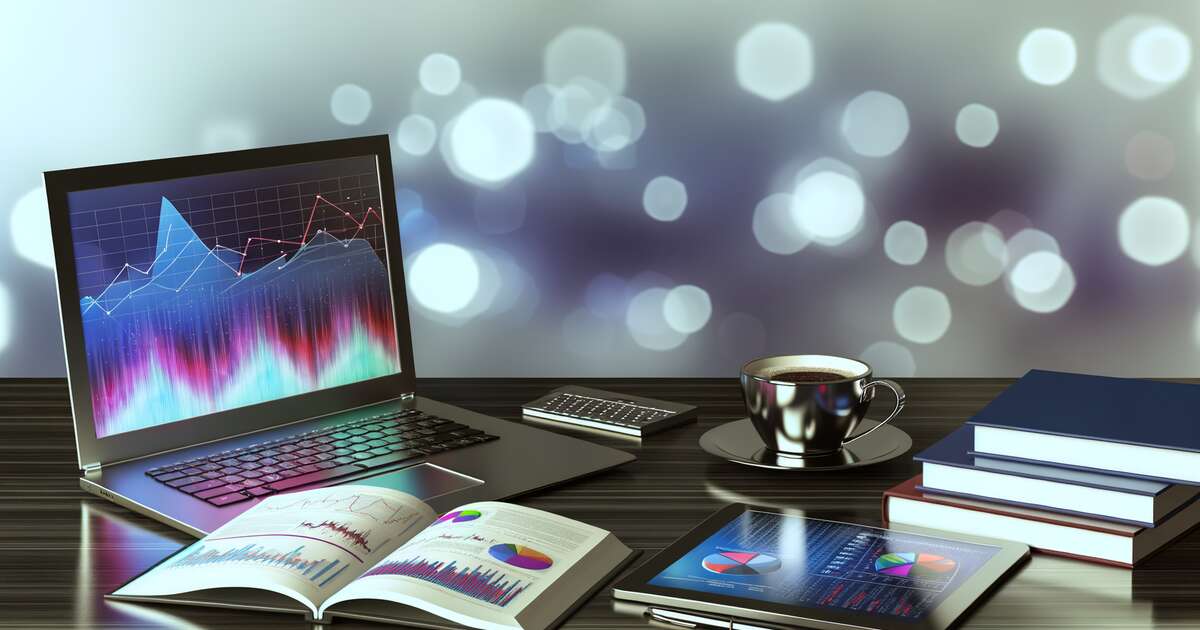
Exploring the world of Revenue Forecasting Models is like unlocking a secret chamber of business success! 🗝️ If you’re aiming to boost your company’s growth, understanding these models can be your golden ticket. But what exactly are these models, and why are they crucial for your business growth?
Revenue forecasting is not just about predicting numbers; it’s a strategic tool that helps in planning and decision-making. From startups to established enterprises, every business can benefit from precise revenue forecasts. In this article, we’ll dive into why these models are indispensable for predicting your future growth accurately and how they can transform uncertainties into actionable insights.
Understanding Basic Revenue Forecasting Models
Understanding basic Revenue Forecasting Models is essential for any business looking to predict and enhance their growth. These models provide a framework to anticipate future revenue, allowing businesses to make informed financial and strategic decisions. But what exactly are they, and why are they so crucial for success?
At its core, revenue forecasting models analyze historical data and market trends to predict future financial outcomes. Whether you’re running a startup or an established company, these models can help gauge the potential returns on investments and adjust business operations strategically. Are you leveraging the right model to align with your business goals?
- Linear Regression Models: Simple yet powerful, these use past revenue data to predict future trends.
- Time Series Analysis: Ideal for businesses with historical data over regular intervals, providing insights into seasonal patterns and growth cycles.
- Machine Learning Techniques: Advanced models that adapt and improve with new data, perfect for dynamic markets and complex datasets.
Choosing the right model hinges on several factors, including the size of your business, the nature of the industry, and data accuracy. By understanding and applying these basic models, you can start to forecast revenue more accurately, driving better decision-making and ultimately, more robust growth. Isn’t it time to harness the power of these models to bolster your business strategy?
How to Apply Quantitative Methods for Revenue Forecasting
Mastering quantitative methods for Revenue Forecasting Models can be a game-changer in accurately predicting future earnings. These methods rely on numerical data and mathematical calculations to provide a clear vision of potential revenue outcomes. But how exactly do you apply these techniques to get the most reliable forecasts?
Firstly, gathering historical sales data is crucial. This data forms the backbone of any quantitative analysis, providing the raw numbers needed to forecast future trends. Have you considered how seasonal trends or economic conditions might factor into your data? Incorporating adjustments for these variables can significantly enhance the precision of your forecasts.
Steps to Implement Quantitative Forecasting
- Collect detailed past sales data.
- Adjust data for seasonality and inflation.
- Apply statistical methods such as regression analysis or time series forecasting.
- Validate the model with recent data to test accuracy.
Implementing these steps helps minimize errors in your revenue projections. Remember, the goal is not just to predict revenue but to do so with enough accuracy that you can confidently make informed financial decisions. Are you ready to upgrade your forecasting model using these quantitative techniques?
Utilizing Qualitative Revenue Forecasting Techniques
Utilizing qualitative Revenue Forecasting Models, particularly in uncertain or rapidly evolving markets, can provide that intuitive edge to businesses. But what makes these models stand apart? Unlike their quantitative counterparts, qualitative techniques rely heavily on expert opinions, market conditions, and trend insights rather than just historical data.
These techniques often include methods like the Delphi method, scenario planning, and market analysis, which allow for a dynamic approach in understanding potential market changes. Aren’t you curious about how these methods can specifically fit into your business strategy?
Consider the power of scenario planning. By examining multiple possible futures, companies can prepare for different outcomes, making them more agile and responsive. This method involves constructing detailed, hypothetical scenarios to explore how changes in the environment might impact future revenue. It’s a way to visualize opportunities and risks and adapt strategies proactively.
Key Advantages of Qualitative Forecasting:
- Flexibility in rapidly changing markets
- Ability to incorporate expert insights
- Enhanced adaptability to new market conditions
Integrating AI and Machine Learning into Revenue Projections
With the evolution of technology, leveraging advanced tools like Artificial Intelligence (AI) and Machine Learning (ML) has become a trendsetter in enhancing how we approach revenue forecasting models. But what exactly does this integration do for your projections? Let’s take a deep dive!
AI and ML algorithms are capable of analyzing large sets of historical data and identifying patterns that may not be obvious to human analysts. This automated analysis helps businesses significantly in predicting future performance with a higher rate of accuracy. From adjusting for seasonal variations to accommodating unpredictable market conditions, the dynamic capabilities of AI can provide a more nuanced and responsive approach to forecasting. Isn’t it fascinating how machine learning can dynamically adapt to new data?
Moreover, integrating AI into Revenue Forecasting Models not only accelerates the process but also reduces the chances of human error. This integration allows for a more systematic approach to scenario planning, enabling decision-makers to prepare better for future fluctuations. Additionally, the predictive power of AI models offers actionable insights, giving businesses the upper hand in strategic planning.
However, it’s crucial to maintain a balanced perspective. The successful integration of AI and ML does require a clear understanding of both the possibilities and the limitations. Are we prepared to upgrade our systems and skill sets to match this new technological advancement in revenue forecasts?
- Enhanced accuracy through pattern recognition
- Efficient data processing reducing human error
- Proactive adaptation to market changes
Best Practices for Accurate Revenue Forecasts
Nailing the accuracy of revenue forecasts is crucial for the successful steering of any business. But how do you ensure your predictions are not just educated guesses but robust, data-driven insights? Here is where best practices come into play! 📊
First and foremost, consistency is key. Establishing a consistent methodology for creating your revenue forecasts ensures that comparisons across different periods or scenarios hold value. Are you conducting your forecasts using the same variables and assumptions? Consistency helps in pinpointing deviations and optimizes strategic adjustments.
Moreover, integrating market and competitor analysis into your revenue forecasting models can dramatically enhance the accuracy. Why not leverage external market dynamics alongside historical data? This informative approach can provide a broader understanding of potential financial outcomes and better prepare you for unforeseen changes in the market.
Finally, regular updates and revisions are indispensable. The business environment is perpetually evolving, and so should your forecasts. By frequently revisiting and adjusting projections based on the latest data, businesses can maintain relevance and improve prediction accuracy. Why settle for outdated predictions when you can optimize with the latest data?
Common Questions
What are the 4 types of forecasting models?
The four primary types of forecasting models are qualitative, time series analysis, causal models, and simulation models. Qualitative forecasting techniques are used when data are limited or non-existent, and they rely on expert opinions or market research. Time series analysis forecasts the future based on past data patterns, and is typically used for short- to medium-term forecasting. Causal models, also known as explanatory models, attempt to account for the causal relationships between variables. Lastly, simulation models are used to recreate and study the behavior of systems to predict future outcomes.
What are the models of income forecasting?
Models of income forecasting typically include econometric models, moving averages, regression analysis, and time series models. Econometric models utilize economic theory to predict future income using mathematical formulas and historical data. Moving averages smooth out income data to identify trends. Regression analysis predicts income based on the relationship between variables and past income data. Time series models, such as ARIMA, exploit patterns found in historical data to project future income levels.
What are the three ways to forecast your revenue?
Three common methods to forecast revenue include historical data analysis, market analysis, and econometric modeling. Historical data analysis involves reviewing past sales data to identify trends and patterns that may continue into the future. Market analysis evaluates external factors such as market demand, competition, and economic environment to forecast future revenue. Econometric modeling combines statistical methods with economic theory to create more comprehensive forecasts that incorporate various external and internal factors influencing revenue.
What are the three types of forecasting?
The three main types of forecasting include trend forecasting, demand forecasting, and causal forecasting. Trend forecasting involves identifying long-term movements in data to predict future outcomes. Demand forecasting estimates future customer demand for a product or service based on historical sales data and market analysis. Causal forecasting looks at the cause-and-effect relationships between different variables, such as economic indicators or consumer sentiment, to predict how changes in these factors will impact the future.
Case Studies: Successful Revenue Forecasting Models in Business
Exploring successful revenue forecasting models can seem like a journey through a vast field of numbers and predictions, but it really positions a business towards incredible growth and stability. Did you know that businesses that invest in robust forecasting models often see a significant improvement in their overall decision-making processes?
One outstanding example is a renowned global retailer that integrated advanced machine learning techniques to forecast demand and optimize stock levels. This adoption not only reduced their operational costs but also enhanced customer satisfaction by ensuring popular products were always available. Another case saw a tech startup use predictive analytics to anticipate market trends and adjust their service offerings accordingly, resulting in a remarkable increase in their market share.
But what makes these Revenue Forecasting Models so effective? It’s their ability to assimilate and analyze vast amounts of data to recognize patterns and predict future outcomes. This accuracy is pivotal—without it, companies might miss out on optimal stock levels or trend adaptations, leading to potential revenue loss. How can your business leverage these insights to avoid similar pitfalls?
- Implementation of AI for dynamic adjustments in forecasting
- Continuous analysis of customer trends and market conditions
- Collaborative planning with all departments to align on forecasted targets
Wrapping Up: Exploring Revenue Forecasting Models for Growth
With the essential insights and qualitative techniques covered, mastering revenue forecasting models feels more achievable than ever! Ready to take your business growth to the next level? By leveraging these best practices and learning from successful case studies, you’re well-equipped to create more accurate and dynamic forecasts. Remember, every bit of precision in forecasting can significantly impact your strategic decisions and ultimately, your business success.
So, why wait? Start integrating advanced tools like AI and Machine Learning to refine your forecasts and stay ahead in the competitive market. Improved revenue forecasting models aren’t just about numbers; they’re about opening doors to new opportunities and achieving greater heights in your business. Let’s put these models to work and watch your growth trajectory soar! 🚀